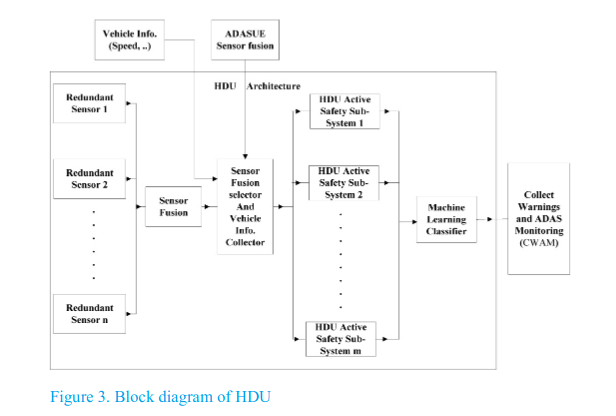
On Board Evaluation System for Advanced Driver Assistance Systems
The evaluation of Advanced Driver Assistance Systems (ADAS including driver assistance and active safety) has increasing interest from authorities, industry and academia. AsPeCSS active safety project concludes that good results in a laboratory test for active safety system design does not necessarily equate to an effective system in real traffic conditions. Moreover, many ADAS assessment projects and standards require physical testing on test tracks (dummy vehicles, pedestrian mannequins.), which are expensive and limit testing capabilities. This research presents a conceptual framework for
DiSGD: A distributed shared-nothing matrix factorization for large scale online recommender systems
With the web-scale data volumes and high velocity of generation rates, it has become crucial that the training process for recommender systems be a continuous process which is performed on live data, i.e., on data streams. In practice, such systems have to address three main requirements including the ability to adapt their trained model with each incoming data element, the ability to handle concept drifts and the ability to scale with the volume of the data. In principle, matrix factorization is one of the popular approaches to train a recommender model. Stochastic Gradient Descent (SGD) has
Motion history of skeletal volumes and temporal change in bounding volume fusion for human action recognition
Human action recognition is an important area of research in computer vision. Its applications include surveillance systems, patient monitoring, human-computer interaction, just to name a few. Numerous techniques have been developed to solve this problem in 2D and 3D spaces. However 3D imaging gained a lot of interest nowadays. In this paper we propose a novel view-independent action recognition algorithm based on fusion between a global feature and a graph based feature. We used the motion history of skeleton volumes; we compute a skeleton for each volume and a motion history for each action
Arabic fake news detection using deep learning
Nowadays, an unprecedented number of users interact through social media platforms and generate a massive amount of content due to the explosion of online communication. However, because user-generated content is unregulated, it may contain offensive content such as fake news, insults, and harassment phrases. The identification of fake news and rumors and their dissemination on social media has become a critical requirement. They have adverse effects on users, businesses, enterprises, and even political regimes and governments. State of the art has tackled the English language for news and
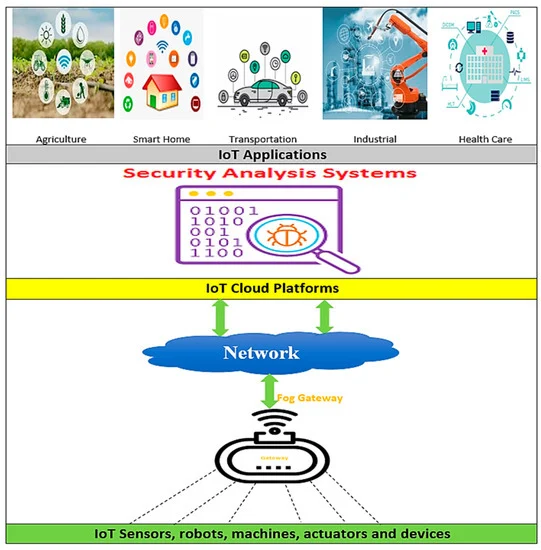
HSAS-MD Analyzer: A Hybrid Security Analysis System Using Model-Checking Technique and Deep Learning for Malware Detection in IoT Apps
Established Internet of Things (IoT) platforms suffer from their inability to determine whether an IoT app is secure or not. A security analysis system (SAS) is a protective shield against any attack that breaks down data privacy and security. Its main task focuses on detecting malware and verifying app behavior. There are many SASs implemented in various IoT applications. Most of them build on utilizing static or dynamic analysis separately. However, the hybrid analysis is the best for obtaining accurate results. The SAS provides an effective outcome according to many criteria related to the
Recognizing Clothing Patterns and Colors for BVI People Using Different Techniques
For blind and visually impaired (BVI) people, it is an arduous task to recognize clothing patterns and colors. It is especially complex to recognize them automatically. This is a highly researched area in image processing. This paper provides BVI with the ability to detect patterns and colors without depending on personal assistance, leading to increasing their confidence. The user first captures an image; then, our system detects colors and patterns. The color model used here is the hue saturation value (HSV). The main colors detected by our system are magenta, cyan, blue, green, red and
Transverse momentum spectra of strange hadrons within extensive and nonextensive statistics
Using generic (non)extensive statistics, in which the underlying system likely autonomously manifests its extensive and nonextensive statistical nature, we extract various fit parameters from the CMS experiment and compare these to the corresponding results obtained from Tsallis and Boltzmann statistics. The present study is designed to indicate the possible variations between the three types of statistical approaches and characterizes their dependence on collision energy, multiplicity, and size of the system of interest. We analyze the transverse momentum spectra pT of the strange hadrons Ks
AutoDLCon: An Approach for Controlling the Automated Tuning for Deep Learning Networks
Neural networks have become the main building block on revolutionizing the field of artificial intelligence aided applications. With the wide availability of data and the increasing capacity of computing resources, they triggered a new era of state-of-the-art results in diverse directions. However, building neural network models is domain-specific, and figuring out the best architecture and hyper-parameters in each problem is still an art. In practice, it is a highly iterative process that is very time-consuming, requires substantial computing resources, and needs deep knowledge and solid
ANN-Python prediction model for the compressive strength of green concrete
Purpose: Utilization of sustainable materials is a global demand in the construction industry. Hence, this study aims to integrate waste management and artificial intelligence by developing an artificial neural network (ANN) model to predict the compressive strength of green concrete. The proposed model allows the use of recycled coarse aggregate (RCA), recycled fine aggregate (RFA) and fly ash (FA) as partial replacements of concrete constituents. Design/methodology/approach: The model is constructed, trained and validated using python through a set of experimental data collected from the
Evaluation of Different Sarcasm Detection Models for Arabic News Headlines
Being sarcastic is to say something and to mean something else. Detecting sarcasm is key for social media analysis to differentiate between the two opposite polarities that an utterance may convey. Different techniques for detecting sarcasm are varying from rule-based models to Machine Learning and Deep Learning models. However, researchers tend to leverage Deep Learning in detecting sarcasm recently. On the other hand, the Arabic language has not witnessed much improvement in this research area. Bridging the gap in sarcasm detection of the Arabic language is the target behind this work. In
Pagination
- Previous page ‹‹
- Page 3
- Next page ››