Controlled alternate quantum walk-based pseudo-random number generator and its application to quantum color image encryption
Pseudo-random number generator (PRNG) are a key component in the design of modern cryptographic mechanisms and are regarded as a backbone element of many modern cryptographic applications. However and in spite of their robustness, quantum computers could crack down PNGR-based systems. Quantum walks, a universal model of quantum computation, have nonlinear properties that make them a robust candidate to produce PRNG. In this paper, we utilize controlled alternate quantum walk (CAQW) to create PRNG. Moreover, we use the presented PRNG mechanism as a component of a new quantum color image
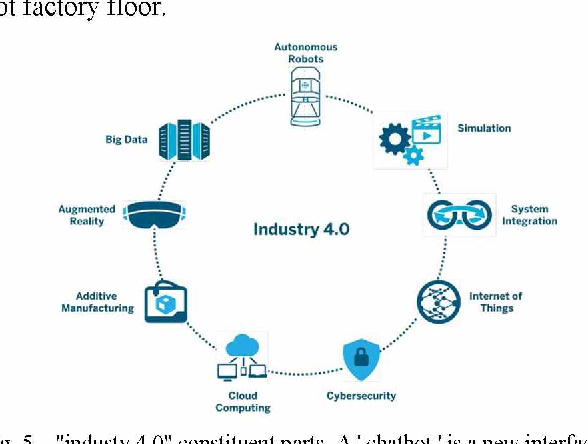
IoT Based AI and its Implementations in Industries
The Internet of Things (IoT) is an Internet revolution that is increasingly used in business, industry, medicine, the economy and other modern information society. IoT, particularly transport, industrial robots and automation systems are supported by artificial intelligence in a wide range of daily implementations with dominant industrial applications. IoT is an interconnected network of physical objects, which enables them to gather and share information, using software, sensor units and network connectivity. In industries; IoT brought about a new revolution in industries. In the field of IoT
Multi projection fusion for real-time semantic segmentation of 3D LiDAR point clouds
Semantic segmentation of 3D point cloud data is essential for enhanced high-level perception in autonomous platforms. Furthermore, given the increasing deployment of LiDAR sensors onboard of cars and drones, a special emphasis is also placed on non-computationally intensive algorithms that operate on mobile GPUs. Previous efficient state-of-the-art methods relied on 2D spherical projection of point clouds as input for 2D fully convolutional neural networks to balance the accuracy-speed trade-off. This paper introduces a novel approach for 3D point cloud semantic segmentation that exploits
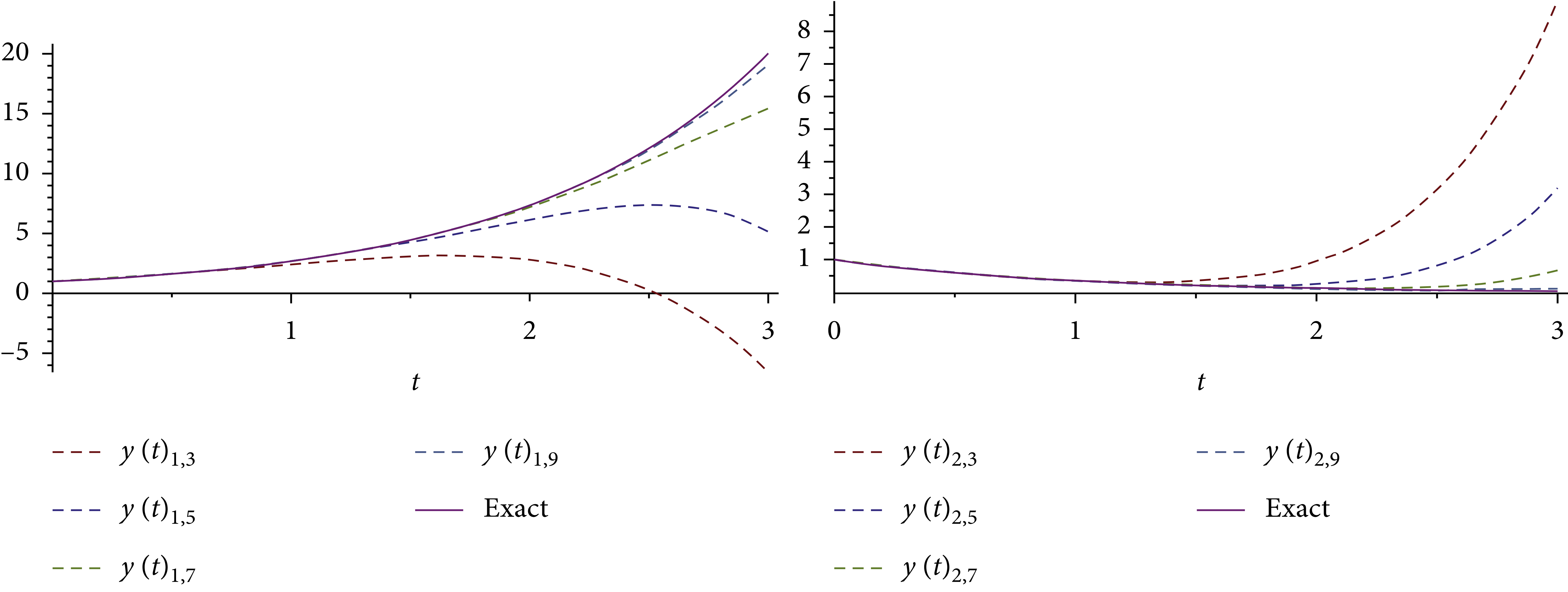
An Analytical Computational Algorithm for Solving a System of Multipantograph DDEs Using Laplace Variational Iteration Algorithm
In this research, an approximation symbolic algorithm is suggested to obtain an approximate solution of multipantograph system of type delay differential equations (DDEs) using a combination of Laplace transform and variational iteration algorithm (VIA). The corresponding convergence results are acquired, and an efficient algorithm for choosing a feasible Lagrange multiplier is designed in the solving process. The application of the Laplace variational iteration algorithm (LVIA) for the problems is clarified. With graphics and tables, LVIA approximates to a high degree of accuracy with a few
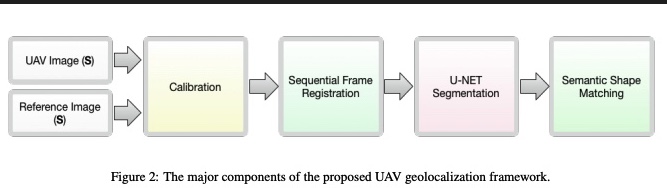
A deep CNN-based framework for enhanced aerial imagery registration with applications to UAV geolocalization
In this paper we present a novel framework for geolocalizing Unmanned Aerial Vehicles (UAVs) using only their onboard camera. The framework exploits the abundance of satellite imagery, along with established computer vision and deep learning methods, to locate the UAV in a satellite imagery map. It utilizes the contextual information extracted from the scene to attain increased geolocalization accuracy and enable navigation without the use of a Global Positioning System (GPS), which is advantageous in GPS-denied environments and provides additional enhancement to existing GPS-based systems
Motion history of skeletal volumes for human action recognition
Human action recognition is an important area of research in computer vision. Its applications include surveillance systems, patient monitoring, human-computer interaction, just to name a few. Numerous techniques have been developed to solve this problem in 2D and 3D spaces. However most of the existing techniques are view-dependent. In this paper we propose a novel view-independent action recognition algorithm based on the motion history of skeletons in 3D. First, we compute a skeleton for each volume and a motion history for each action. Then, alignment is performed using cylindrical
AraVec: A set of Arabic Word Embedding Models for use in Arabic NLP
Advancements in neural networks have led to developments in fields like computer vision, speech recognition and natural language processing (NLP). One of the most influential recent developments in NLP is the use of word embeddings, where words are represented as vectors in a continuous space, capturing many syntactic and semantic relations among them. AraVec is a pre-Trained distributed word representation (word embedding) open source project which aims to provide the Arabic NLP research community with free to use and powerful word embedding models. The first version of AraVec provides six
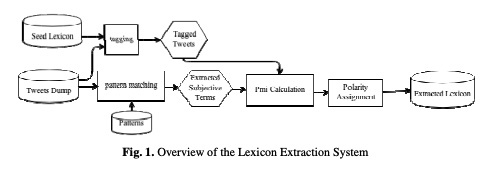
A fully automated approach for Arabic slang lexicon extraction from microblogs
With the rapid increase in the volume of Arabic opinionated posts on different social media forums, comes an increased demand for Arabic sentiment analysis tools and resources. Social media posts, especially those made by the younger generation, are usually written using colloquial Arabic and include a lot of slang, many of which evolves over time. While some work has been carried out to build modern standard Arabic sentiment lexicons, these need to be supplemented with dialectical terms and continuously updated with slang. This paper proposes a fully automated approach for building a
Exploiting neural networks to enhance trend forecasting for hotels reservations
Hotel revenue management is perceived as a managerial tool for room revenue maximization. A typical revenue management system contains two main components: Forecasting and Optimization. A forecasting component that gives accurate forecasts is a cornerstone in any revenue management system. It simply draws a good picture for the future demand. The output of the forecast component is then used for optimization and allocation in such a way that maximizes revenue. This shows how it is important to have a reliable and precise forecasting system. Neural Networks have been successful in forecasting
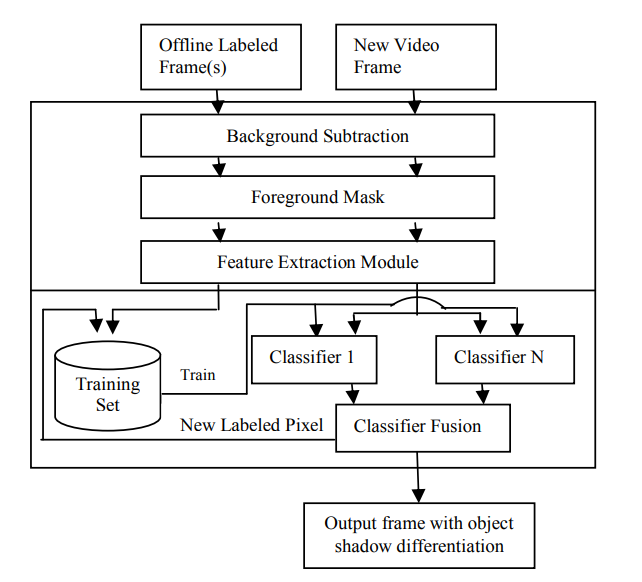
A semi supervised learning-based method for adaptive shadow detection
In vision-based systems, cast shadow detection is one of the key problems that must be alleviated in order to achieve robust segmentation of moving objects. Most methods for shadow detection require significant human input and they work in static settings. This paper proposes a novel approach for adaptive shadow detection by using semi-supervised learning which is a technique that has been widely utilized in various pattern recognition applications and exploits the use of labeled and unlabeled data to improve classification. The approach can be summarized as follows: First, we extract color
Pagination
- Previous page ‹‹
- Page 23
- Next page ››